In the relentless pursuit of artificial intelligence (AI) advancement, organizations often focus on model architectures, algorithm optimization, and computational power. Yet, a critical component remains under-appreciated: the efficiency of data retrieval across diverse formats. While AI research investments have reached record highs, with companies spending billions on GPUs and compute infrastructure, relatively little attention is given to the way data is retrieved, indexed, and processed. The result is a widening efficiency gap—one that costs organizations not just in time but in direct financial impact.
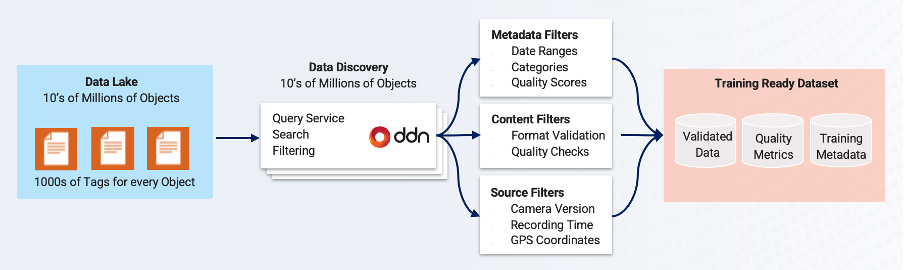
AI engineers struggle with delays in accessing the right training datasets, retrieval inefficiencies when searching through massive repositories, and integration challenges when pulling from multiple, disparate data formats. A McKinsey report estimated that data scientists spend up to 80% of their time simply wrangling and preparing data, rather than training models (McKinsey & Company, 2022). Data analysts often grapple with repositories containing thousands of labeled objects, such as PDFs or videos, each annotated with multiple labels. For instance, the Visual Genome dataset comprises approximately 100,000 images, each richly annotated with numerous labels, creating a detailed visual knowledge base.
Similarly, Google’s Open Images dataset offers a collection of nine million URLs to images, annotated with labels spanning over 6,000 categories.
Navigating these extensive datasets to locate a specific subset of data becomes a daunting task, often leading to significant delays in data processing and analysis.
Financial Services: Accelerating Investment Research and High-Frequency Trading
The financial sector is one of the most aggressive adopters of AI, leveraging machine learning for portfolio management, algorithmic trading, fraud detection, and risk analysis. Hedge funds and banks ingest enormous amounts of real-time market data, alternative data sources, and historical financial records to build predictive models that determine investment strategies. Yet, despite AI’s potential to unlock untapped alpha, data access bottlenecks remain a major hurdle.
High-frequency trading (HFT) firms, for example, rely on executing trades at speeds measured in microseconds. Delayed data access leads to missed opportunities, and in a competitive environment, latency differentials of even a fraction of a millisecond can mean the difference between profit and loss. According to research from MIT, traders using automated AI-driven strategies execute trades in times as low as 10 microseconds, yet the ability to retrieve and act on market data still represents a serious challenge (MIT Sloan School of Management, 2023). AI models that can efficiently process financial documents, such as SEC filings, earnings call transcripts, and analyst reports, give firms an informational advantage, but only if retrieval mechanisms can support the scale and complexity of this data.
A failure to optimize retrieval times doesn’t just slow down trades—it reduces returns. Firms that address this efficiency gap are able to process and act on market signals up to 10x faster, reducing risk exposure and improving profitability. In a world where trading is measured in milliseconds and AI-driven arbitrage dominates, financial institutions simply cannot afford retrieval inefficiencies.
Life Sciences: Accelerating Drug Discovery and Precision Medicine
Nowhere is AI’s impact on society clearer than in the life sciences industry. AI is revolutionizing genomics, medical imaging, and pharmaceutical research, yet slow data retrieval threatens to stall innovation. Biotech companies rely on massive datasets—genomic sequences, clinical trial data, molecular simulations, and high-resolution scans—that must be accessed, processed, and analyzed in near real-time to develop new drugs and treatments. The faster researchers can retrieve and process data, the quicker they can generate insights that lead to life-saving medical breakthroughs.
A study by Deloitte found that the average cost to develop a new drug exceeds $2.6 billion, and much of that cost stems from inefficiencies in research and clinical trials (Deloitte, 2023). One of the largest bottlenecks is the time required to retrieve and correlate relevant datasets. AI-powered drug discovery platforms must integrate structured and unstructured data from diverse sources, including patient records, protein folding simulations, and real-time trial results. If this retrieval process is sluggish, it extends the entire R&D timeline, delaying critical treatments from reaching patients.
Faster data retrieval in this domain translates directly to economic impact. Pharmaceutical companies with accelerated AI-driven discovery pipelines are bringing drugs to market years ahead of competitors, capturing billions in revenue while improving patient outcomes. The ability to instantly retrieve, analyze, and correlate biological datasets determines which firms lead the next generation of biotech innovation.
Autonomous Vehicles: Processing the World in Real Time
The self-driving car revolution is entirely dependent on AI’s ability to process vast amounts of sensor data in real time. Each autonomous vehicle generates as much as 4 terabytes of data per hour, sourced from cameras, LiDAR, radar, and telemetry systems (Intel, 2023). AI models must analyze this data stream with near-instantaneous retrieval to make split-second driving decisions. A single delay in accessing critical information can mean the difference between a safe maneuver and a collision.
Yet, current retrieval models struggle under the sheer weight of autonomous vehicle datasets. Traditional indexing methods fail to keep up with the demands of AI-driven decision-making in dynamic environments. A research study from Stanford University highlighted that data latency greater than 100 milliseconds introduces significant risks in autonomous navigation, as it impairs the ability of AI models to react to fast-changing road conditions (Stanford AI Lab, 2023).
By eliminating retrieval delays and enabling real-time sensor fusion, self-driving systems gain the responsiveness they need to function safely in chaotic environments. The economic implications are profound. Autonomous vehicle manufacturers who solve the retrieval problem first will achieve faster regulatory approvals, mass-market adoption, and a dominant position in an industry projected to be worth $556 billion by 2026 (Allied Market Research, 2023).
Recent benchmarking results demonstrate that DDN’s optimized data access solutions can outperform the most widely used object platforms by a staggering 100x factor, dramatically reducing retrieval times and accelerating AI workflows. This is made possible by a completely new and novel architecture designed to introduce ultra-fast data warehousing and analytics capabilities natively into our keyspace. By eliminating traditional inefficiencies, this architecture dramatically reduces latency and significantly increases rates for data identification, enabling AI models to access and act on relevant information instantaneously. Common AI data preparation tasks, such as joins, aggregations, and filtering operations, require rapid retrieval and indexing of distributed datasets, a challenge that traditional architectures struggle to meet at scale. Our approach enables SQL-like operations, including joins and unions, to execute with near-zero delays, ensuring that massive datasets can be prepared, cleaned, and analyzed without bottlenecks.
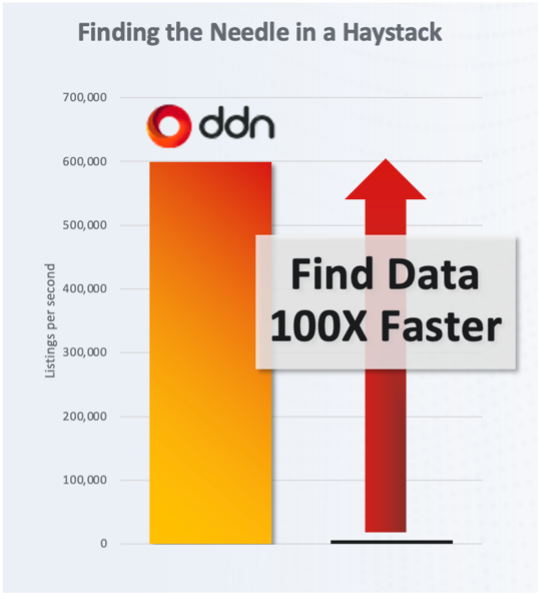
Frameworks like Apache Spark, Dask, and Presto are widely used for AI-driven analytics, but their performance is often hindered by slow data retrieval. While Spark excels in distributed computing, its efficiency is directly tied to how quickly it can access and process relevant datasets. Our architecture optimizes this interaction, ensuring that ETL pipelines, feature engineering processes, and AI model training are not stalled by outdated storage mechanisms. The impact is transformative: financial institutions can execute trades with real-time intelligence, biotech firms can shorten drug discovery cycles, and autonomous vehicle companies can process exponentially more sensor data in real time, enhancing safety and operational efficiency. Across industries, the organizations that optimize data retrieval will unlock superior AI performance, lower operational costs, and establish an enduring competitive edge.
Conclusion: The Fastest Wins—Everyone Else Loses
There’s a silent arms race happening in AI. It’s not about GPUs or billion-parameter models—it’s about how fast organizations can retrieve, process, and act on data. The ability to instantly retrieve structured and unstructured data from any source, at any scale, is the defining factor in AI’s next evolution. Whether in finance, life sciences, autonomous driving, or large-scale machine learning, those who master data retrieval will achieve breakthroughs that others cannot.
AI doesn’t wait. Neither should you.